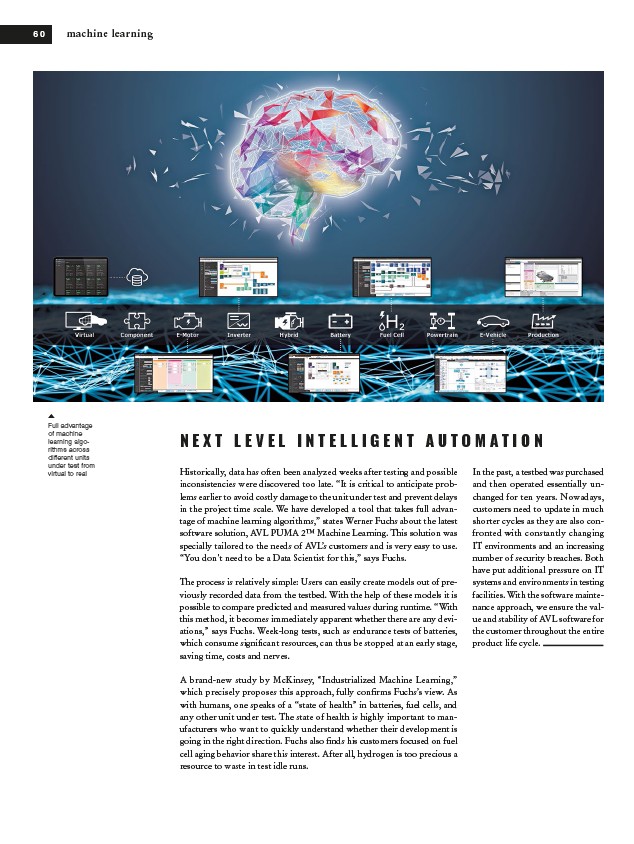
N E X T L E V E L I N T E L L I G E N T A U TO M AT I O N
Full advantage
of machine
learning algorithms
across
different units
under test from
virtual to real Historically, data has often been analyzed weeks after testing and possible
inconsistencies were discovered too late. “It is critical to anticipate problems
earlier to avoid costly damage to the unit under test and prevent delays
in the project time scale. We have developed a tool that takes full advantage
of machine learning algorithms,” states Werner Fuchs about the latest
software solution, AVL PUMA 2™ Machine Learning. This solution was
specially tailored to the needs of AVL’s customers and is very easy to use.
“You don't need to be a Data Scientist for this,” says Fuchs.
The process is relatively simple: Users can easily create models out of previously
recorded data from the testbed. With the help of these models it is
possible to compare predicted and measured values during runtime. “With
this method, it becomes immediately apparent whether there are any deviations,”
says Fuchs. Week-long tests, such as endurance tests of batteries,
which consume significant resources, can thus be stopped at an early stage,
saving time, costs and nerves.
A brand-new study by McKinsey, “Industrialized Machine Learning,”
which precisely proposes this approach, fully confirms Fuchs’s view. As
with humans, one speaks of a “state of health” in batteries, fuel cells, and
any other unit under test. The state of health is highly important to manufacturers
who want to quickly understand whether their development is
going in the right direction. Fuchs also finds his customers focused on fuel
cell aging behavior share this interest. After all, hydrogen is too precious a
resource to waste in test idle runs.
In the past, a testbed was purchased
and then operated essentially unchanged
for ten years. Nowadays,
customers need to update in much
shorter cycles as they are also confronted
with constantly changing
IT environments and an increasing
number of security breaches. Both
have put additional pressure on IT
systems and environments in testing
facilities. With the software maintenance
approach, we ensure the value
and stability of AVL software for
the customer throughout the entire
product life cycle. �
6 0 machine learning