AVL in R&D: Prediction of vehicle battery lifetime thanks to AI & Federated Learning
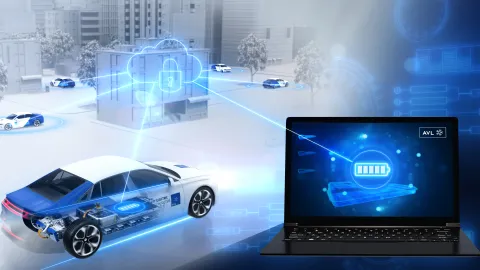
How long does an e-car last? That depends to a large extent on the lifetime of the battery. Using artificial intelligence or more specifically federated learning approaches, this can be predicted and subsequently optimized. A global neural network is trained with a wealth of decentrally stored data from different vehicle fleets - without actually sharing the data or compromising data protection. The AI model is then available as a basis for calculating the remaining useful lifetime. The calculation method was developed by AVL together with the Institute of Automotive Technology at the Technical University of Munich (TUM).
Graz, Austria, November, 2023: A long life for car batteries. This is something that not only car drivers are concerned about. The UN is also calling for a minimum shelf life for e-car batteries. According to the proposal, these should still have 80 percent of their original capacity after five years or 100,000 kilometers in use. In order to optimize the lifetime of batteries, it is necessary to determine the current state and predict future behavior. This can be done with the help of machine learning. Here, lots of data is used to generate empirical values. By using the federated learning method, a model is trained with large, decentrally stored data from different vehicle fleets. "Each fleet trains its own neural network. The knowledge gained is then transferred to a global model. Hence, no one has to access an OEM's original data," says Annalena Belnarsch, a development engineer at AVL. She developed the method together with the Institute of Automotive Technology at TUM and tested it using 50,000 training data samples from the lab. One scenario: "For example, we distributed a data set over ten fleets. Compared to training one neural network per fleet, we were able to estimate the battery lifetime much more accurately thanks to Federated Learning. On average, the error value was reduced by 32 percent."
The more data the better: How OEMs can improve their battery operation strategy
As a general rule for model training: The more, the better. Even smaller fleets with fewer data sets contribute to better predictability - but also benefit from it – while maintaining a very high level of data protection. The more trained neural networks exist to feed the 'central model' can be fed, the more successfully manufacturers can supply their battery management systems with updated models and thus optimize their operating strategy. Incidentally, this also benefits vehicle owners. With the help of AI-based battery analysis, malfunctions can be detected at an early stage. In this way, vehicles can be serviced preventively. This can subsequently contribute to a longer lifetime of the battery or a higher residual value in the event of a possible resale. Considering that a battery costs approx. 150 euros per kWh, precise calculations can be made.
"One of the biggest challenges in training AI models for battery aging prediction is generating a large data set, as the vehicle data required for this includes sensitive personal or operational information. One solution to this is federated learning, in which all vehicle data remains local to the respective fleet operator and yet an aging model can be trained collaboratively without having to upload one's own data to a server and thus risk data privacy violations or data leaks," says Thomas Kröger, Research Associate at the Institute of Automotive Technology at TUM.
"The mutual exchange between science, research and industry is driving the necessary paradigm shift toward sustainable mobility. The Technical University of Munich has been associated with AVL List GmbH in this regard for many years, and their joint work is dedicated, among other things, to making electrified driving even more attractive to people," says Prof. Markus Lienkamp, head of the Institute of Automotive Technology at TUM and a board member of the VDI Association for Automotive and Transport Engineering .
The full study, "Collaborative Training of a Deep Neural Network for the Lithium-Ion Battery Aging Prediction with Federated Learning," can be read in the current issue of the eTransportation Journal.
With more than 12,200 employees, AVL is one of the world's leading mobility technology companies for development, simulation and testing in the automotive industry and in other sectors such as rail, shipping and energy. Based on extensive in-house research activities, AVL delivers concepts, technology solutions, methodologies and development tools for a greener, safer and better world of mobility and beyond.
AVL supports international partners and customers in sustainable and digital transformation. The focus here is on the areas of electrification, software, AI and automation. AVL also supports companies in energy-intensive sectors on their way to green and efficient energy generation and supply.
AVL's passion is innovation. Together with an international network of experts spanning 90 locations with more than 50 competence and development centers worldwide, AVL is driving the future of mobility. In 2024, the company generated a turnover of 2.03 billion euros, of which 11% was invested in R&D activities.
For more information: www.avl.com
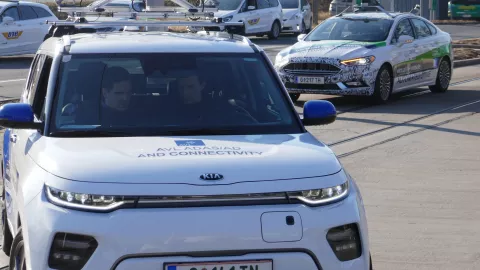
- EN,
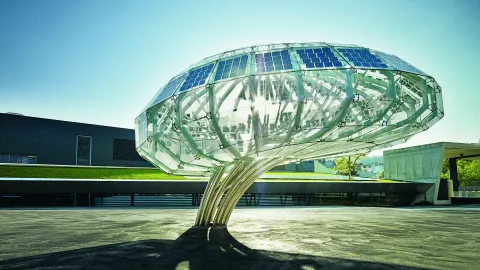
- EN,
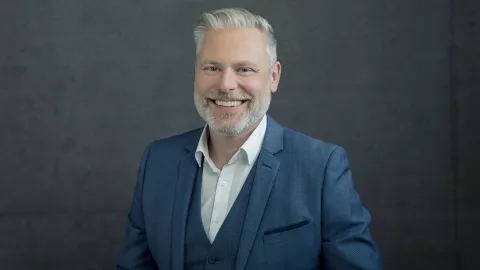
- EN,
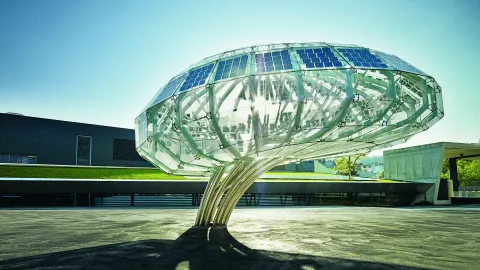
- EN,
Stay up-to-date as a journalist with our latest press releases concerning company updates, research projects, our latest developments, and more.
Download our fact sheet to get a comprehensive overview about what we do as well as our latest company figures.