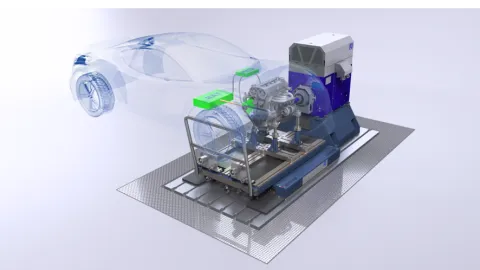
Automotive Industry is moving rapidly towards environmental friendly propulsions system. Hybrid powertrains are capable to lower CO2 consumption and pollutant emissions. A typical approach is to find the optimum hybrid layout and strategy, by performing certain technical design tasks in office simulation directly followed by vehicle prototype tests on the chassis dyno and road. This leads to a high number of complex prototype vehicles, overload on chassis dynos, time-consuming road test and finally to tremendous costs.To overcome this time- and cost drivers we invented an innovative approach using an engine testbed as bridging element between office simulation and vehicle testing. The Engine Test system get extended with real-time vehicle simulation capabilities including repeatable driver behavior. Complemented with simulated electric hybrid components like e-Motor, Battery, as well as electric consumers and most important hybrid control functionalities simulated within an HCU model.The simulated HCU and its freely definable operating strategy make it possible to vary typical hybrid operating scenarios like electric boosting or electric recuperating within defined areas. Using a DoE approach including model creation, interdependencies between the amount of IC-Engine load point moving direction sweet spot areas - and emissions, as well as fuel consumption, can be made clear and transparent. This enables to find optimum conditions and derive most effective operating strategies. On top of real measured emissions and fuel consumption, numerous additional Key Performance Indicators like battery current throughput or battery SOC – load dependencies can be investigated to get the most robust and reliable control strategy in an objective way. This increase the HCU maturity level in the early stages and consequently decreases the development effort on cost-intensive prototype vehicles.